The rise of AI-powered tools is transforming marketing—offering unprecedented efficiency, scale, and automation. But can AI truly replace the strategic and creative force of human marketers?
The Forrester report ‘Frontline Marketer vs. Machine’ explores this dynamic. On the plus side, AI marketing tools excel in automation and data‑driven insights. However, they still fall short in key areas: creativity, strategic alignment, and brand governance.
How does AI and human marketing performance differ?
AI marketing automation has reshaped the way teams work—eliminating bottlenecks in asset reformatting, metadata tagging, and workflow execution. These tools analyze enormous volumes of data at unmatched speeds. This enables real-time optimization of digital ad placements and boosts ROI. From campaign personalization to performance tracking, AI in digital marketing delivers measurable productivity gains.
While impressive, AI lacks the emotional intelligence and cultural context to elevate content from functional to truly compelling. The gap between scale and storytelling remains a human domain.
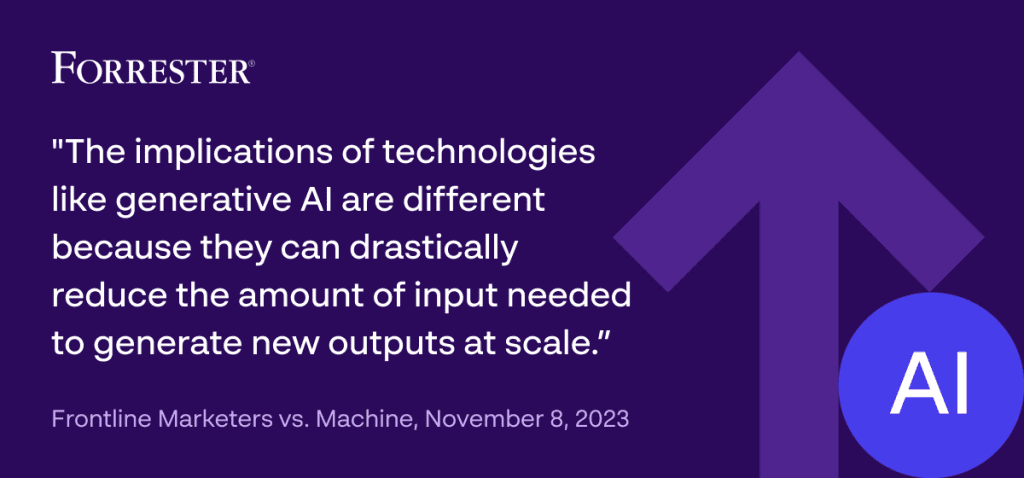
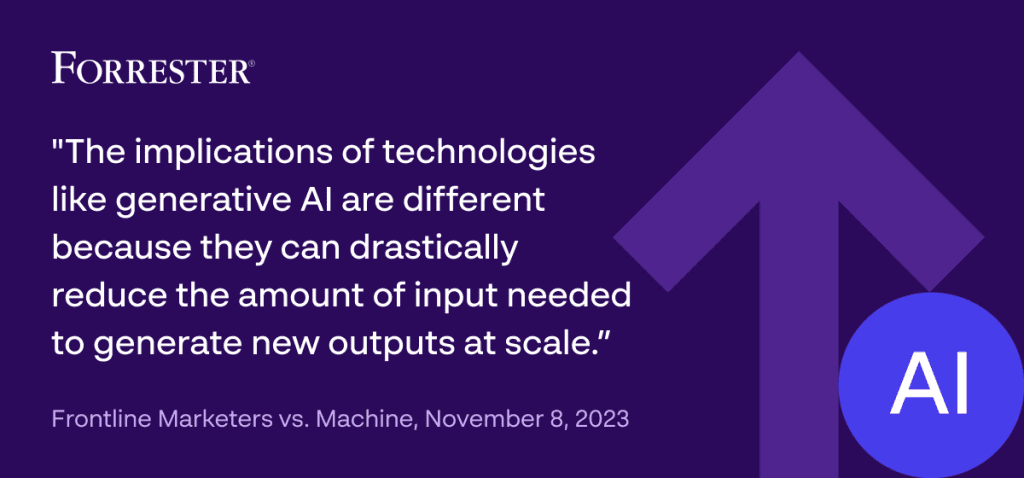
The challenges of using AI in marketing
Despite the speed and volume advantages, AI introduces real challenges for marketers. Generative AI for marketing can churn out content rapidly. Ensuring that content reflects brand voice, emotional nuance, and local relevance requires human intervention. AI still struggles with sarcasm, humor, cultural sensitivity, and message alignment—raising risks for brand perception and trust.
“Frontline marketing leaders must anticipate AI’s potential to replace headcounts, roles, accountabilities, or activities […] ” – Forrester report, November 8, 2023
Even the best AI tools for marketing are only as effective as the data they’re trained on. If inputs are biased or outdated, the output can mislead rather than inform—undermining both strategy and ROI.
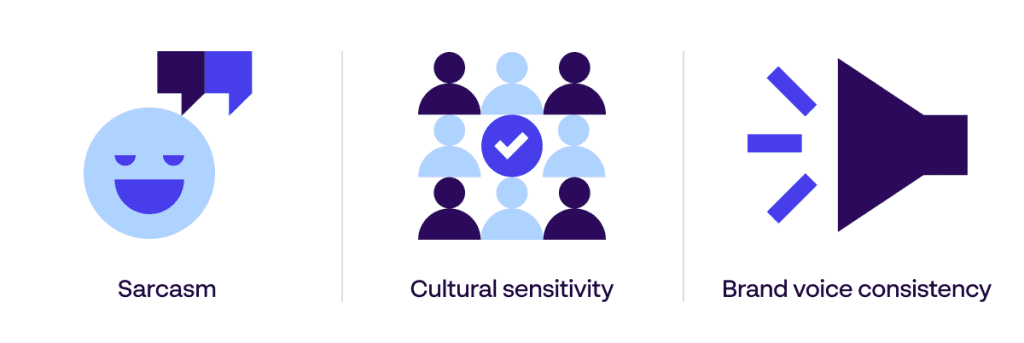
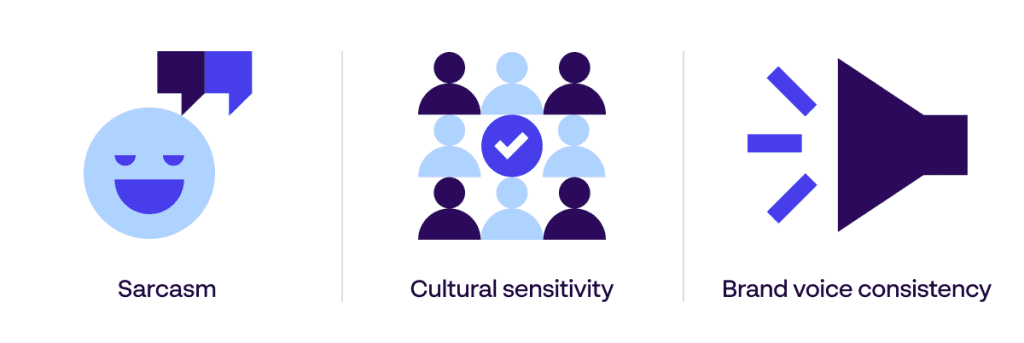
Can AI replace human creativity?
Creativity is still the greatest limitation for AI content marketing. While these tools can support content generation, they rely on patterns and historical data—not original thinking. That’s why brand-defining storytelling, emotional resonance, and campaign innovation remain areas where human marketers shine.
“Forrester recommends using generative AI to enhance, not replace, human efforts in the short term.” – Forrester report, November 8, 2023
Where marketers continue to outperform AI
The advancement of AI marketing solutions falls short in replacing marketers. Strategic thinking, contextual awareness, and brand governance sits with your people. Interpreting complex customer needs, responding in real-time to emerging situations, and maintaining brand consistency across regions require human expertise.
“Because AI is destined to become an indispensable, trusted enterprise coworker, frontline marketing leaders must ensure their human workers are equipped with the right technology skills.” – Forrester report, November 8, 2023
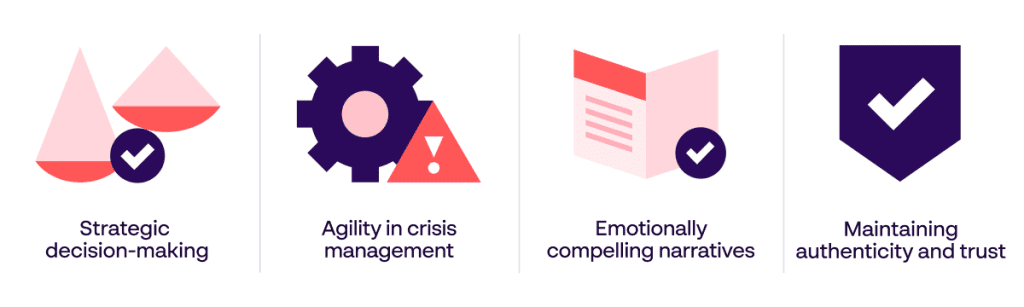
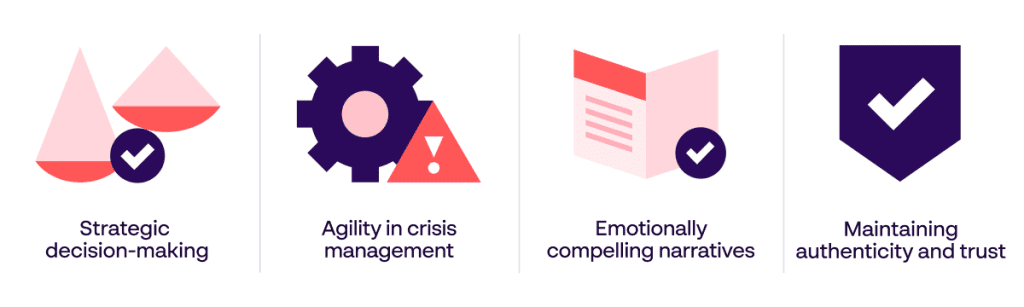
How to integrate AI in marketing without losing control
AI should be integrated as a support layer—not a substitute. Use it to automate routine tasks, surface insights, and accelerate production. But always apply human oversight for quality control, message alignment, and brand integrity.
Building AI fluency among your people ensures that AI tools enhance your output. They can’t replace the people who drive strategy. As Forrester puts it: “Enhance, not replace, human efforts.”
Brand consistency and control: The limits of automation
AI can support brand governance by automating compliance checks, applying content templates, and maintaining formatting standards. But consistency is more than appearance—it’s about tone, relevance, and brand authenticity. Over-relying on AI risks producing content that’s accurate but emotionally flat or misaligned across regions.
“Frontline marketing leaders must get ahead of the trend and define the specific ways in which humans and technologies should be driving productivity in their frontline organizations.” – Forrester report, November 8, 2023
Risks and rewards for global marketing teams
AI offers tremendous benefits in marketing automation—improved speed, reduced production costs, and scalable personalization. But global teams must be mindful of the trade-offs: diluted brand voice, ethical risks, and reduced creative agility. Marketers must manage AI with clear guardrails and guide it with human insight to unlock its full value.
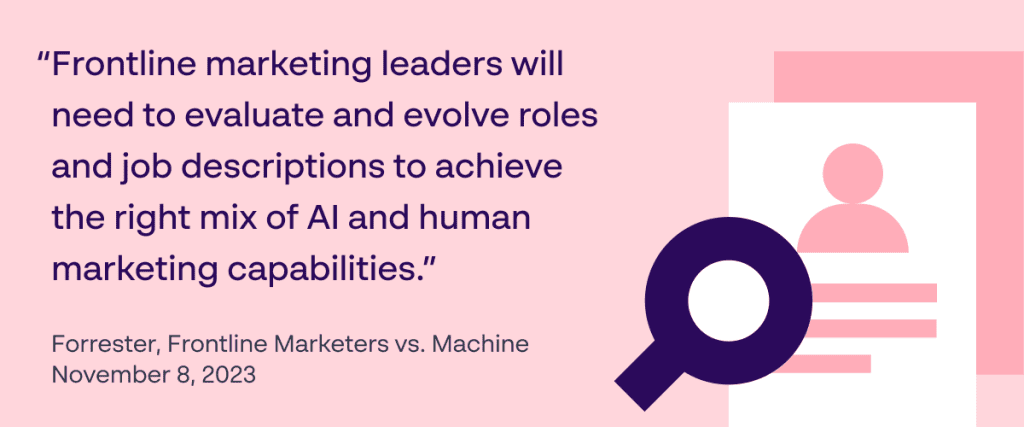
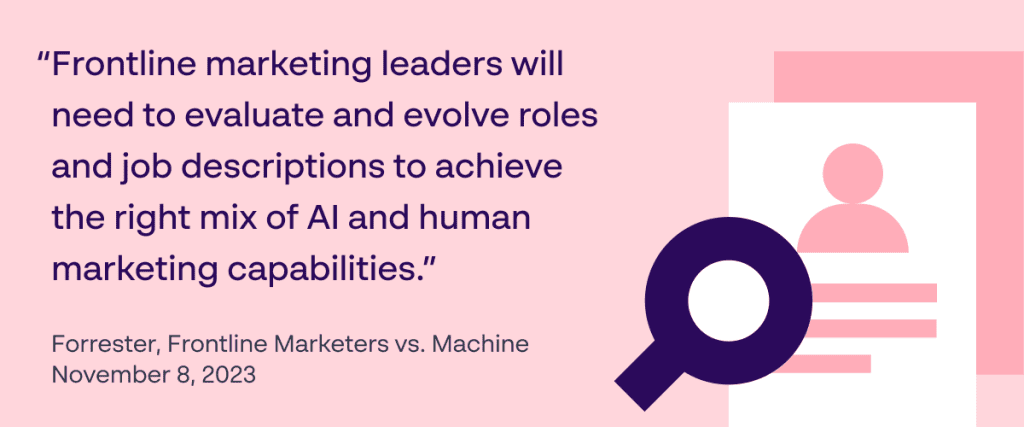
The future: AI‑augmented, not AI‑replaced
AI is here to stay—and it’s helping lighten the load on marketing operations. Using AI in marketing teams unlocks powerful efficiency and insight. Yet human marketers remain essential. People bring qualities that cannot be automated—empathy, originality, and judgment.
The future of marketing is a partnership. AI marketing tools accelerate execution, while human marketers ensure creativity, strategy, and brand control stay intact.
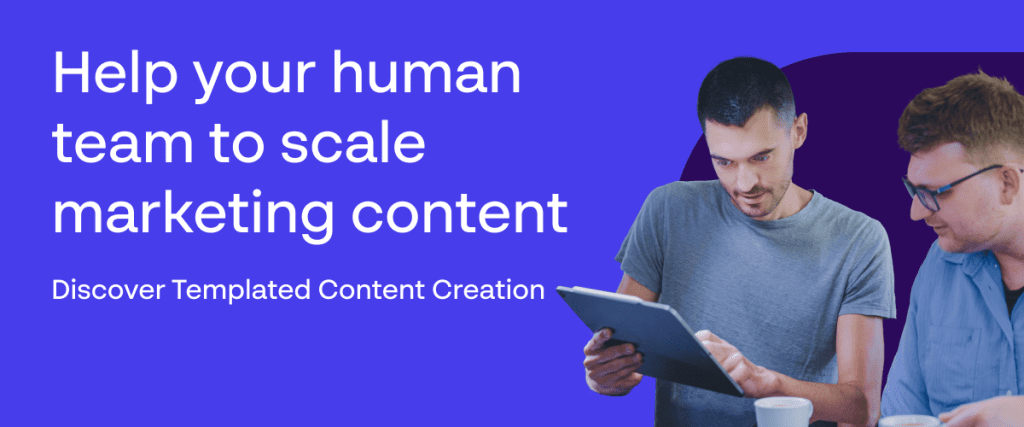
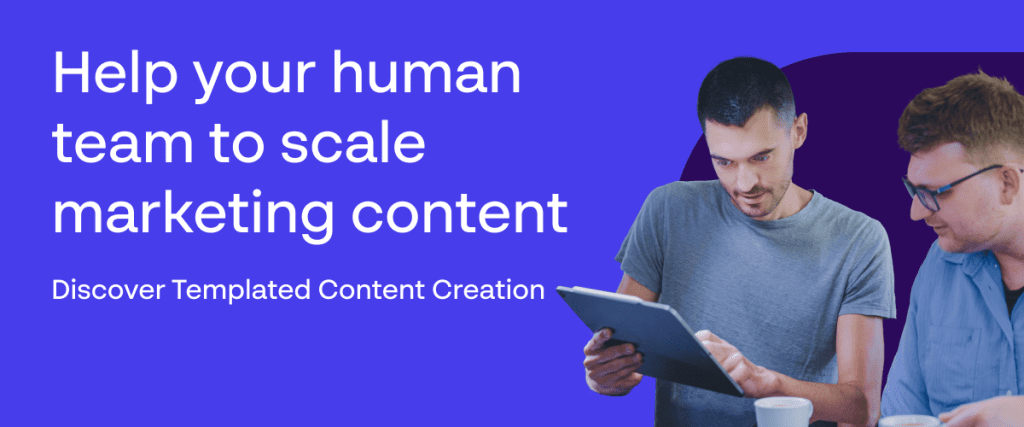