You don’t have to look far to feel the air of AI-scaremongering. Robots coming for our jobs, AGI (General Artificial Intelligence) surpassing human ability, reaching the point of ‘Singularity’ – the hypothesis that AI will become smarter than people and then be uncontrollable, and the end of humankind as we know it. Movies like The Terminator, Minority Report, and Ex Machina have Hollywoodified Earth’s surrender to technology for years now.
When you ask corporate leaders about the risks AI present to their organisations, you tend to get similar answers based on similar themes:
- What about copyright and intellectual property?
- What about job displacement and human capital?
- Are we at the start of a machine-led world?
- Are we at risk of machine intelligence replacing human intelligence?
- How do we compete against infallible machine intelligence?
- How do we move fast enough to mitigate the risk of being out-run?
- Who wins in the end – us, our competitors, new entrants, or AI generally?
- Who can be trusted to regulate this new world?
Each of these questions can be unravelled to create opportunity alongside risk, but many leaders currently find it hard to differentiate. There is so much noise. There is still a talent gap – it’s estimated we need 10x the computer science graduates we have today in order to meet the hiring plans already announced by major software companies. The rapidity of change is increasing faster than existing operational models, such as fiscal years or quarterly reporting, were designed for.
This means, in all likelihood, that we must look elsewhere for a rational and unencumbered view. Let’s look at what academics have reached agreement on.
Academics don’t routinely agree with each other but for the past decade thought leaders from the biggest and best technology education institutions (including Massachusetts Institute of Technology [MIT], University of Oxford, Stanford University, Indian Institute of Technology, National University of Singapore, etc) have settled on the Three Pitfalls of AI as being Privacy, Replication, and Bias.
Privacy
Defined as the ability of an individual or group to seclude either themselves (or information about themselves), and thereby be selective in what they express, privacy is a phrase we’re all familiar with. The domain of privacy partially overlaps with security, which can include the concepts of appropriate use and protection of information.
But the abuse of privacy can be more abstract. Consider the (existing) patents that connect social media profiles with dynamic pricing in retail stores. The positioned use case is usually a discount presented to a shopper because the retailer’s technology knows – from learned social media data – that they are likely to buy if presented with a coupon or offer. This feels win-win for both parties and therefore the data shared feels like a transactional exchange rather than a privacy intrusion.
However, where that same technology can be used to inflate the price of a prescription for antidepressants – because the data tells the retailer’s system that the shopper is likely struggling with their mental health – it quickly becomes apparent that the human cost of privacy abuse could be very high indeed.
Privacy is considered one of the three pitfalls of AI because data (and so often personal data) is so intrinsically linked to machine intelligence’s success. The conversation around who owns that data, how that data should / should not be used, how to educate people about the importance of data, and how to give users more control over the data has been happening in pockets (but far from all) of society for a long time. As AI advances, it’s widely acknowledged that this area has to evolve in tandem.
Replication
The inability to replicate a decision made by Al – often referred to as a ‘black box’ – occurs when programmers and creators or owners of technologies do not understand why their machine makes one decision and not another.
Replication is essential to proving the efficacy of an experiment. We must know that the results a machine produces can be used consistently in the real world, and that they didn’t happen randomly. Using the same data, the same logic, and the same structure, machine learning can produce varying results and / or struggle to repeat a previous result. Both of these are problematic – and can be particularly troublesome when it comes to algorithms trained to learn from experience (reinforcement learning) where errors become multiplied.
The ‘black box’ approach is often excused by claiming IP protection or ‘beta’ status of products. But the prolonged inability to interrogate, inspect, understand, and challenge results from machines leads to an inability for humans to trust machines. Whether that’s confusion about how a lender has credit-scored your mortgage application, or something even more serious like not being able to prove that a prospective employer has used machine learning to discriminate against a candidate.
Replication is considered one of the three pitfalls of AI because we need to know we can trust AI. For us to trust it we need to be able to understand it. To be able to understand it we need to be able to replicate it.
Bias
“A tendency, inclination, or prejudice toward – or against – something or someone” is how bias is usually defined. Today, Google has more than 328m results when you search for “AI bias”. Unfortunately AI and bias seem to go hand-in-hand with a new story about machine intelligence getting it (very) wrong appearing daily.
As the use of artificial intelligence becomes more prevalent, its impact on personal data sensitive areas – including recruitment, the justice system, healthcare settings, and financial services inclusion – the focus on fairness, equality, and discrimination has rightly become more pronounced.
The challenge at the heart of machine bias is, unsurprisingly, human bias. As humans build the algorithms, define training data, and teach machines what good looks like, the inherent biases (conscious or otherwise) of the machines’ creators become baked-in.
Investigative news outlet ProPublica has shown how a system used to predict reoffending rates in Florida incorrectly labelled African American defendants as ‘high-risk’ at nearly twice the rate it mislabeled white defendants. The system didn’t invent this bias – it extrapolated and built upon assumptions programmed by its creators.
Technologists and product leaders like to use the acronym GIGO – ‘Garbage In, Garbage Out’ – and it absolutely applies here. When we train machines to think, all of the assumptions we include at the beginning become exponentially problematic as that technology scales.
Replication is considered one of the three pitfalls of AI because technology is often spoken of as being a great ‘leveller’, creating opportunities, and democratising access. But so long as AI bias is as bad as, or worse than, human bias, we will in fact be going backwards – with large sections of society disadvantaged.
Responding to AI’s challenges
Each of these Three Pitfalls of AI are serious and they have attracted a lot of attention – including from the leaders of the very companies at the forefront of AI’s development and evolution. When more than 1,100 CEOs wrote the now-infamous open letter calling for a halt to AI development they were essentially asking for time for humans to catch up and think about the possible consequences of our actions.
There are further questions about regulation – with lawmakers struggling to keep up with the rate of change. Trust of politicians remains stubbornly low globally and the public is also hesitant to trust a small group of technology company billionaires with what realistically could be existential threats to parts of how we live today. But self-regulation is where we are at currently and that means it comes down to individual technology creators to build responsibly and ethically.
At Papirfly we have defined an Ethical Charter that governs what technology we build, how we build it, and the ethical parameters within which we build it. In Part 4 of this series we’ll share our Ethical Charter and demonstrate how it works in our company.
Watch our on-demand webinar
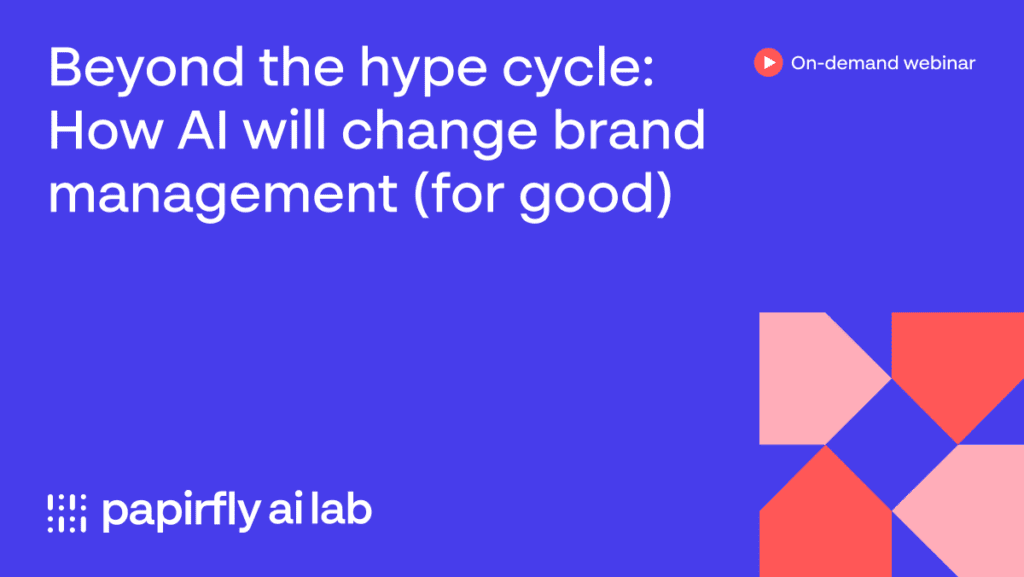
Table of contents: